If you’re a data software engineer equipped with data and software skills, the field of Deep Learning presents abundant opportunities. Deep Learning, recognized as the fastest-growing information technology, is widely adopted by companies worldwide. It comprises techniques that predict outputs from layered inputs.
Undoubtedly, securing a Deep Learning job is highly desirable for every data or computer engineer due to its perks and prestige. However, obtaining such a job isn’t a given; companies seek candidates with expertise in science and data skills. Before applying for a data scientist position in Deep Learning, it’s crucial to enhance your skills and knowledge in the field.
Remember, your success in securing a job hinges on the interview, so honing your interview skills is essential. Whether you’re a fresher or a seasoned professional, interview nerves are common. However, knowing the types of questions typically asked in a Deep Learning interview can significantly benefit job candidates.
If you’re seeking Deep Learning interview questions, you’re in the right place. In this article, we’ll provide everything you need to prepare for your upcoming interview. Peruse the following list of top Deep Learning interview questions to ensure you’re well-prepared.
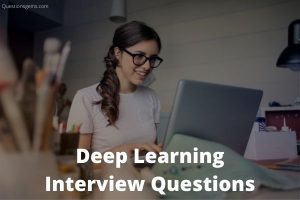
Deep Learning Interview Questions
Q1.Is It Ok To Connect From A Layer 4 Output Back To A Layer 2 Input?Ans-Yes, this can be done considering that layer 4 output is from previous time step like in RNN. Also, we need to assume that previous input batch is sometimes- correlated with current batch.
Q2.What Is An Auto-encoder?Ans-An autoencoder is an autonomous Machine learning algorithm that uses backpropagation principle, where the target values are set to be equal to the inputs provided. Internally, it has a hidden layer that describes a code used to represent the input.–Some Key Facts about the autoencoder are as follows:-It is an unsupervised ML algorithm similar to Principal Component AnalysisIt minimizes the same objective function as Principal Component AnalysisIt is a neural networkThe neural network’s target output is its input.
Q3.What Is A Model Capacity?Ans-Ability to approximate any given function. The higher model capacity is the larger amount of information that can be stored in the network.
Q4.What Is An Autoencoder?Ans-Autoencoder is artificial neural networks able to learn representation for a set of data (encoding), without any supervision. The network learns by copying its input to the output, typically internal representation has smaller dimensions than input vector so that they can learn efficient ways of representing data. Autoencoder consist of two parts, an encoder tries to fit the inputs to an internal representation and decoder converts internal state to the outputs.
Q5.What Is Data Normalization And Why Do We Need It?Ans-Data normalization is very important preprocessing step, used to rescale values to fit in a specific range to assure better convergence during backpropagation. In general, it boils down to subtracting the mean of each data point and dividing by its standard deviation.
Q6.Weight Initialization In Neural Networks?Ans-Weight initialization is a very important step. Bad weight initialization can prevent a network from learning. Good initialization can lead to quicker convergence and better overall error. Biases can be generally initialized to zero. The general rule for setting the weights is to be close to zero without being too small.
Q7.Why Is Zero Initialization Not A Recommended Weight Initialization Technique?Ans-As a result of setting weights in the network to zero, all the neurons at each layer are producing the same output and the same gradients during backpropagation.The network can’t learn at all because there is no source of asymmetry between neurons. That is why we need to add randomness to weight initialization process.
Q8.What Is The Role Of The Activation Function?Ans-The goal of an activation function is to introduce nonlinearity into the neural network so that it can learn more complex function. Without it, the neural network would be only able to learn function which is a linear combination of its input data.
Q9.Why Are Deep Networks Better Than Shallow Ones?Ans-Both shallow and deep networks are capable of approximating any function. For the same level of accuracy, deeper networks can be much more efficient in terms of computation and number of parameters. Deeper networks are able to create deep representations, at every layer, the network learns a new, more abstract representation of the input.
Q10.What Is A Dropout?Ans-Dropout is a regularization technique for reducing overfitting in neural networks. At each training step we randomly drop out (set to zero) set of nodes, thus we create a different model for each training case, all of these models share weights. It’s a form of model averaging.
Q11.What Is Weight Initialization In Neural Networks?Ans-Weight initialization is one of the very important steps. A bad weight initialization can prevent a network from learning but good weight initialization helps in giving a quicker convergence and a better overall error. Biases can be generally initialized to zero. The rule for setting the weights is to be close to zero without being too small.
Conclusion:
Here are some of the most commonly asked Deep Learning questions you’ve been seeking. We trust you’ve found everything you needed, and armed with this list, you’ll be well-prepared for your interview. Thank you for visiting, and best of luck with your upcoming interview!